How Ai Is Revolutionizing The Healthcare Industry
Artificial intelligence (AI) is rapidly transforming healthcare by making medical care faster, more accurate, and more personalized. AI systems can analyze vast amounts of data – from medical images and genetic sequences to electronic health records – to assist clinicians in diagnosing diseases, tailoring treatments, and predicting outcomes. For example, AI algorithms now help doctors interpret X-rays and MRIs, pinpoint the best cancer therapies, and even sift through millions of research articles. These tools are reducing costs and human error while enabling 24/7 patient support. As one comprehensive review notes, AI “optimizes operational efficiency, streamlines administrative tasks, and improves patient flow” in hospitals. In this article, we explore the many ways AI is revolutionizing healthcare – from diagnostics to drug discovery – and examine real-world examples, current challenges, and future directions.
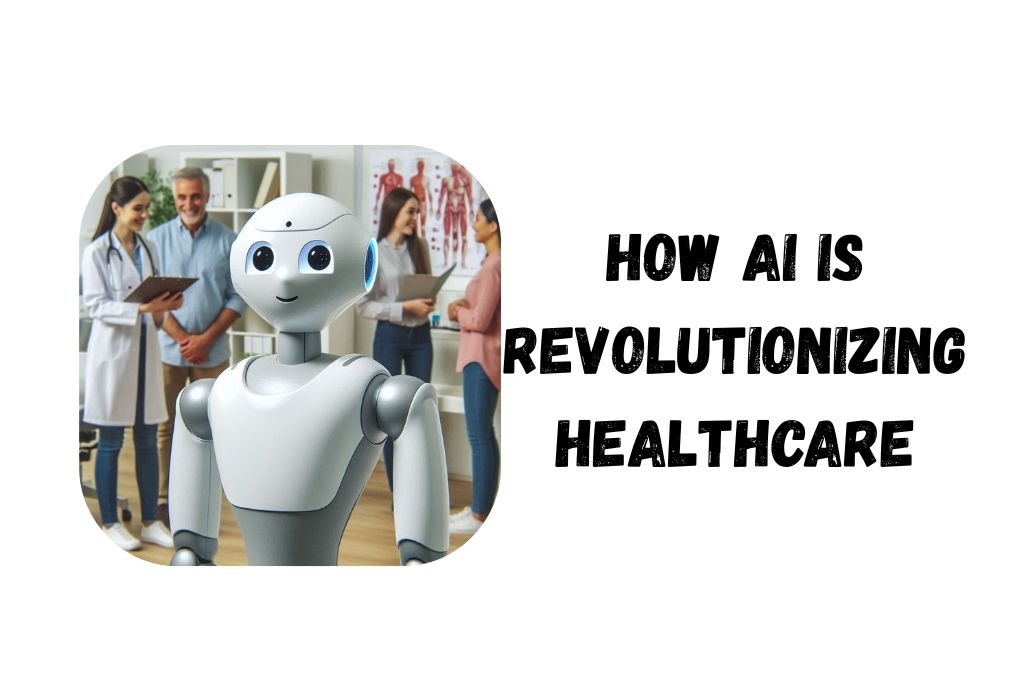
AI in Diagnostics and Medical Imaging
AI is enhancing diagnostics by accurately interpreting medical images and tests. Modern deep-learning systems can detect subtle patterns invisible to the human eye, helping doctors catch diseases earlier and more reliably. For instance:
- Radiology (X‑rays, CT, MRI): AI can flag pneumonia, fractures, tumors and other findings on chest X-rays and scans. In one study, an FDA‑cleared AI tool achieved a 0.976 area-under-curve (AUC) score on chest X‑rays, and when used alongside physicians it significantly improved detection of abnormalities – non-radiologist doctors aided by AI matched radiologist-level accuracy. This “narrowed the gap” between generalists and specialists, speeding up diagnosis.
- Ophthalmology (retina): AI systems are now approved to autonomously screen for diabetic eye disease. In the U.S., three FDA-cleared AI devices (IDx-DR, EyeArt, AEYE Health) can analyze retinal images and detect diabetic retinopathy without a human reader. These tools allow more patients to be tested cheaply and frequently (even in remote clinics), improving early treatment and preventing blindness.
- Pathology and Dermatology: AI can also classify skin lesions or examine biopsy slides. Several algorithms match or exceed dermatologists in spotting melanoma and other skin cancers, and AI-based microscopes can highlight cancer cells on biopsy samples. (For example, IBM Watson Health and various startups are developing AI for pathology workflows.) These tools are still being validated clinically, but early results show AI-as-co-pilot can speed up diagnosis.
By automating routine image analysis and lab interpretation, AI frees clinicians to focus on complex cases. It also reduces human error: for example, radiologists can miss abnormalities on X-rays about 30% of the time, whereas AI assistance greatly lowers that miss rate. Ultimately, AI-driven diagnostics aim to provide faster, more reliable readings – especially in underserved areas with few specialists.
AI for Personalized Medicine
AI is ushering in a new era of personalized medicine, where treatment is tailored to each patient’s unique biology. Instead of one-size-fits-all drugs, doctors can use AI to match therapies to a patient’s genetic profile, imaging “fingerprints,” and other data. Key developments include:
- Cancer Treatment Optimization: AI systems analyze imaging features (radiomics), genomics and patient history to predict which therapies will work. For example, in a 2024 study an AI-powered radiomics tool predicted cancer patients’ response to immunotherapy with 89% accuracy, compared to only 60% accuracy using conventional methods. Such precision means patients get the right drugs faster (and avoid ineffective treatments). Big companies like Siemens Healthineers, RaySearch and RadNet are already deploying AI platforms to tailor radiation doses and drug plans based on tumor imaging data.
- Genomic Analysis: Companies and research centers are harnessing AI to interpret DNA and RNA data. For instance, Illumina (a gene-sequencing giant) recently partnered with AI firm Tempus to integrate AI into clinical genomics. They use machine learning to sift through patients’ molecular profiles and identify the best treatments. The goal is to “route every patient to the optimal therapy based on molecular insights,” beyond just cancer – including cardiology and neurology. Similarly, Pfizer and others are leveraging AI-driven pharmacogenomics: as Pfizer notes, AI helps “tailor drug delivery to individual genetic makeup,” moving away from one-size-fits-all prescribing.
- Risk Stratification: AI analyzes genetic data and health records to flag high-risk individuals before disease strikes. For example, machine-learning models can identify people with genetic markers or family history that put them at higher risk for diabetes, heart disease or rare conditions. This enables proactive care – more frequent monitoring, preventive medication or lifestyle interventions. As one review explains, AI’s ability to “analyze vast datasets” lets clinicians detect conditions early and “tailor treatment to individual patient profiles”.
In practice, personalized AI tools are already changing care. Some hospitals use AI-based clinical decision-support to scan patient data and suggest customized treatment paths. Over time, as more genomic and multi-modal data become standard, AI will increasingly design personalized care – from cancer therapy to drug dosing – for every patient.
AI in Drug Discovery and Development
Traditional drug discovery is extremely slow and costly (fewer than 12% of new drugs make it through trials). AI is accelerating drug development by automating and optimizing each stage:
- Target Identification and Screening: AI models can scan databases of molecules and predict how they interact with disease targets. For example, DeepMind’s AlphaFold – an AI that predicts protein structures – is revolutionizing this field. The latest AlphaFold 3 can predict the 3D shapes and interactions of biomolecules with unprecedented accuracy (50–100% better than previous methods). Such accuracy helps scientists rapidly identify which proteins to target and design drugs that fit them. Companies (like DeepMind’s sister firm Isomorphic Labs) are already collaborating with pharma to apply AlphaFold to real drug design challenges.
- Drug Design and Optimization: AI algorithms suggest chemical structures that could treat a disease. For instance, Pfizer has leveraged AI to shorten development time: its AI platform helped design the first oral COVID-19 antiviral in record time. (Pfizer credits AI with accelerating vaccine and drug discovery). Startups like Insilico Medicine and BenevolentAI use generative models to propose novel compounds or repurpose existing drugs, dramatically shrinking the number of candidates to test.
- Simulation and Trials: AI-driven in silico models can simulate how drugs behave in the body, reducing reliance on animal models. Additionally, AI helps select patients for clinical trials (finding those most likely to respond) and predicts adverse effects. This improves success rates – an ongoing goal given the historically low 12% approval rate.
In short, AI’s data-crunching power is making drug pipelines faster and smarter. Rather than years of trial-and-error chemistry, researchers can use AI to predict which molecules will succeed, saving time and money. As one industry article notes, “artificial intelligence is poised to transform the drug discovery landscape”. Over the coming years we expect AI to churn out new drug candidates at an unprecedented pace, bringing treatments for complex diseases to market faster than ever.
AI in Robotic Surgery
AI is dramatically advancing robotic-assisted surgery, improving precision and expanding capabilities. Surgical robots (such as Intuitive Surgical’s da Vinci system) let surgeons perform minimally invasive operations with tiny, articulating instruments. With AI support, these systems become even more powerful:
Figure: Robotic surgical arms (da Vinci system) with precision instruments. AI helps guide these systems for minimally invasive procedures.
- Precision and Control: Modern surgical robots translate a surgeon’s hand movements into microscopic motions. For example, the da Vinci Xi robot uses high-resolution cameras and flexible arms to work inside the body through tiny incisions. As a U.S. Army press release describes, “small articulating instruments demonstrate the precision and strength of the da Vinci Xi surgical system,” enabling complex surgeries with millimeter accuracy. AI assists by filtering tremors, optimizing movements, and augmenting the surgeon’s view (e.g. highlighting nerves or tumors on-screen).
- Human–Robot Partnership: Currently, robotic surgery is a team effort: the surgeon plans and guides, and the robot executes flawlessly. As one expert analogized, it’s “like power steering in a car” – the surgeon steers, and the robot follows the hand precisely. This “shared control” setup already improves patient outcomes (less blood loss, faster recovery) and is being refined constantly. AI enables the robot to anticipate surgeon actions and enforce safety limits (for instance, preventing movements that could cut important tissue). Over time, machine learning will allow robots to learn from expert surgeons’ movements.
- Towards Autonomy: Research labs are pushing toward more autonomous surgery. In a 2024 breakthrough, Johns Hopkins engineers used imitation learning (training by watching) to teach a da Vinci robot new skills. The AI watched videos from thousands of surgeries and learned to manipulate a needle, lift tissue, and suture, performing these tasks “as skillfully as human doctors”. This represents a huge step toward robots that can perform routine surgical tasks independently, under human supervision. Experts envision a future where robots handle standard procedures or assist scarce surgeons in remote areas: one researcher noted AI robots could “fill a critical role” given the global surgeon shortage.
In summary, robotic surgery today combines human expertise with machine precision. AI is enhancing these systems by providing real-time guidance, improved ergonomics, and even partial autonomy. As robotic intelligence advances, more procedures may become possible robotically, making surgery safer and more widely accessible.
AI-Powered Virtual Health Assistants
AI is also transforming patient interactions through virtual assistants and chatbots. These tools provide instant, 24/7 support to patients and help healthcare providers streamline communication. Notable examples include:
- Symptom Triage and Information: AI chatbots on websites or apps can evaluate a patient’s symptoms and advise on next steps. For instance, digital health platforms like Babylon Health offer chatbots that collect a patient’s history and symptoms, then suggest possible diagnoses or whether to see a doctor. Similarly, many hospitals now have online assistants to answer basic medical questions or direct patients to the appropriate clinic. By instantly giving guidance (e.g., “Do I need urgent care for this headache?”), these bots improve access to care and reduce unnecessary ER visits.
- Chronic Disease Support: Virtual assistants help patients manage long-term conditions. A prominent example is Molly by Sensely, an AI nurse avatar that checks in with chronic patients (like diabetics or heart failure patients) by phone or app. Molly asks about symptoms, reminds patients to take medications, and alerts clinicians if readings are concerning. This continuous monitoring keeps patients on track with their care plans and catches issues early. For instance, a diabetic patient might get daily prompts to measure blood sugar, with the AI adjusting reminders based on their data history.
- Administrative Assistance: Many clinics use AI assistants to handle routine tasks. Chatbots can schedule appointments or send reminders, reducing no-shows. For example, Zydus Hospitals in India use an AI chatbot to autonomously book and confirm patient appointments, greatly cutting down administrative workload. Voice assistants (like Amazon Alexa or Google Assistant) are also gaining “health skills” – e.g., Mayo Clinic’s Alexa app provides first-aid advice on demand.
These virtual agents ease pressure on healthcare systems by handling simple queries and routine follow-ups, allowing human staff to focus on critical care. They also engage patients outside the clinic: 24/7 availability and personalized interactions (tailored to a patient’s history) improve adherence and satisfaction. Overall, AI-powered chatbots and voice assistants are making healthcare more interactive and accessible for patients and providers alike.
AI in Hospital Operations and Administration
Beyond patient care, AI is streamlining hospital workflows and management behind the scenes. By automating routine work and optimizing processes, AI is making healthcare more efficient:
- Scheduling and Patient Flow: Hospitals use AI to forecast demand and schedule staff. Machine-learning models analyze historical admissions, surgeries, and seasonal trends to predict how many patients will arrive on a given day. This lets administrators adjust staffing levels and reduce wait times. For example, AI-driven tools can alert managers when emergency room crowding is likely, so extra nurses or beds can be allocated proactively. In fact, a major review notes that in hospital management “AI optimizes operational efficiency, streamlines administrative tasks, and improves patient flow and scheduling”. In practice, some hospitals have cut appointment no-shows and ER delays by using predictive analytics to overbook or shuffle appointments.
- Automating Administration: Routine paperwork and coordination can be a huge drag on providers. AI systems are now automating many of these tasks. For instance, natural language processing is being used to auto-code medical billing and insurance claims based on patient records. Several companies (like Nuance Communications and Ambience Healthcare) offer AI “scribes” that listen to doctor-patient conversations and draft clinical notes in real time. These tools have made documentation a “fundamentally reversed” burden – doctors at one cancer center reported gaining 2–3 hours per day back by using an AI scribe. Automating transcription and data entry frees clinicians from clerical work, improving efficiency and reducing costs.
- Resource and Supply Chain Management: Hospitals also apply AI to manage supplies and equipment. Smart inventory systems track usage of medications, PPE, and implants, automatically reordering items when stocks run low. In pharmacies, AI can double-check prescriptions for interactions. During crises, predictive models help plan resource allocation: for example, AI models have been used to predict oxygen and ventilator needs during COVID-19 surges. By optimizing logistics, AI helps hospitals cut waste and ensure critical resources are available when needed.
By integrating AI into administrative systems, hospitals can operate more smoothly and at lower cost. Studies have shown that an AI framework for scheduling and flow can reduce wait times and improve patient throughput. In one example, an AI project cut outpatient MRI no-shows by automatically overbooking slots based on predicted attendance. Overall, AI is making healthcare administration smarter – reducing paperwork, improving coordination, and ultimately allowing care teams to concentrate on patients.
Predictive Analytics and Disease Prevention
AI’s ability to predict future events is transforming preventive medicine and population health. By mining health data, AI can forecast who will get sick and help stop disease before it starts:
- Patient Risk Prediction: AI models analyze electronic health records, wearable data and even social determinants to flag individuals at high risk of disease. For example, deep learning can combine lab tests, imaging and genomics to predict the onset of conditions like diabetes, heart attack, or kidney failure months or years in advance. One review found that AI predictive analytics “predicts disease progression, optimizes treatment plans, and enables personalized medicine by facilitating early detection”. In practice, this means doctors can intervene earlier – increasing monitoring, prescribing preventive medication, or recommending lifestyle changes for those flagged at risk.
- Treatment Outcome Forecasting: Beyond risk, AI can predict how a patient will respond to a specific therapy. We saw above that AI radiomics could forecast immunotherapy success. Similarly, algorithms are being trained on clinical trial data to predict drug side effects or efficacy in individual patients. By knowing in advance which patients are likely to benefit, clinicians can tailor treatment plans and avoid trial-and-error prescriptions. This makes care more efficient and reduces harm.
- Outbreak Detection and Public Health: On a population level, AI analyzes health trends and mobility data to forecast epidemics. For instance, companies like BlueDot and HealthMap use AI to detect disease clusters from news and airline data; BlueDot famously alerted public health officials to the emerging COVID-19 outbreak faster than official channels in late 2019. The WEF notes that AI could help bridge healthcare gaps for the 4.5 billion people lacking essential services. In future pandemics, AI-driven epidemiological models will guide resource allocation (e.g. where to send vaccines or field hospitals) and enable real-time interventions. By spotting outbreaks early and predicting their course, AI helps prevent widespread illness.
In the consumer space, wearables (like smartwatches) feed data into AI apps that alert users to potential problems (irregular heartbeats, low oxygen, etc.) so they can seek care early. AI also powers health recommender systems – for example, apps that analyze diet, exercise and genetics to suggest preventive health measures for chronic diseases. Altogether, predictive analytics is shifting healthcare from reactive to proactive: we can spot disease signals before symptoms appear and take action to avert serious outcomes.
Limitations and Ethical Concerns
While AI holds tremendous promise, several challenges and ethical issues remain. Key concerns include:
- Data Privacy & Security: AI systems require large amounts of patient data (images, records, genetics). Safeguarding this sensitive information is paramount. Health data breaches or misuse could violate privacy rights. Regulations like HIPAA in the U.S. aim to protect patient information, but as one expert notes, robust encryption and governance are still needed to prevent unauthorized access. Hospitals and tech firms must ensure AI algorithms comply with data-protection laws and that patients consent to how their data are used.
- Algorithmic Bias and Equity: AI is only as good as the data it learns from. If training datasets are unrepresentative, AI can perpetuate or worsen health disparities. For example, a model trained mostly on data from one ethnic group might give inaccurate results for others. As a review highlights, “AI systems are only as unbiased as the data they learn from; biased datasets can lead to healthcare disparities”. It is crucial to test AI tools on diverse populations and to correct any biases. Otherwise, AI could amplify inequities (e.g. by systematically under-diagnosing certain groups).
- Transparency and Trust: Many AI models (especially deep learning) are “black boxes” – it’s hard to understand why they make a certain prediction. This lack of transparency can make clinicians uneasy about trusting the AI. Ethical guidelines emphasize the need for explainability: doctors and patients should know the rationale behind an AI recommendation. Without explainability, it’s difficult to assign accountability if an AI system errs. This also complicates regulatory approval, since regulators require evidence of safety and validity.
- Regulation and Validation: Unlike traditional medical devices, AI software is evolving continuously. Ensuring these tools are safe and effective requires new regulatory approaches. AI algorithms must undergo rigorous clinical trials or real-world testing. Currently, some AI diagnostic tools have faced recalls or overhyped claims. Ethical use demands ongoing model validation and clear protocols for deployment. Medical societies stress that AI should augment – not replace – human judgment. In other words, AI should assist clinicians (e.g. “AI copilot”), who remain ultimately responsible for patient care.
- Technical and Practical Barriers: Deploying AI also faces technical hurdles – integrating AI tools into electronic health record systems, ensuring user-friendly interfaces, and training staff to use them. Poor integration or workflow design can impede adoption. Additionally, small or underfunded hospitals may lack the infrastructure (computing power, data storage) to run advanced AI systems, widening the gap between rich and poor healthcare settings.
In summary, AI in healthcare carries ethical imperatives. Stakeholders must address privacy, fairness, accountability, and governance as these technologies spread. By doing so, the healthcare community can “harness the full potential of AI while safeguarding patient welfare”. Transparency, diverse data, and clear regulation will be key to earning public trust.
Future Outlook
Looking ahead, AI’s role in healthcare will only grow. The convergence of AI with genomics, wearables, and telemedicine heralds a future of truly data-driven, patient-centered care. For example, next-generation AI might continuously monitor our health via smart sensors and alert us to problems instantly. Combining genomics with AI could enable personalized vaccines and cures for rare diseases. Robotics may extend to remote surgery or rehabilitation, overcoming current surgeon shortages.
Industry forecasts support this momentum. For instance, the market for AI tools in healthcare (including generative AI) is projected to expand rapidly (estimated to reach billions of dollars by 2030). Major institutions like the World Economic Forum note that AI could help achieve global health goals by extending care to underserved populations. Researchers are already working on “multimodal” AI that fuses imaging, blood tests, and genomics to detect diseases like cancer at their very earliest stages. Meanwhile, ongoing advances in AI explainability and safety aim to make these tools more reliable and ethically sound.
In conclusion, artificial intelligence is revolutionizing healthcare on multiple fronts. From smarter diagnostics and personalized treatments to streamlined operations and preventative care, AI is reshaping the medical landscape. As clinicians and technologists tackle the current challenges (data privacy, bias, validation), the promise of AI remains vast: safer surgeries, targeted therapies, and more accessible healthcare for all. The future of medicine will be increasingly AI-augmented – transforming how diseases are detected, prevented and cured, and ultimately improving patient outcomes across the globe.